Prediction of breast cancer using tools of machine learning techniques
Abstract
Author(s): Madhumita Pal, Smita Parija* and Ganapati Panda
Objective: Machine learning techniques have been shown to support multiple medical prognoses. The purpose of this article is to compare some machine learning techniques to compare the diagnosis of breast cancer (cancerous and non-cancerous) using the inputs from five supervised machine learning approaches through the different feature selections to get a correct result.
Materials and methods: This study included 683 cases of breast cancer, four hundred and forty-four being benign and two hundred and thirty-nine malignant; the studied data were taken from the UCI machine learning repository. Ten models for machine learning were evaluated and only five were selected from the correlation matrix (SVC, logistic regression, random forests, XGBoost or K-NNs).
Results: Random forests and the K-NNs model predict the most significant true positives among the five techniques. In addition, SVC and RFs models predict the most significant number of true negatives and the lowest number of false negatives. The SVC obtains the highest specificity of 96% and the XGB obtains the lowest specificity of 92.3%.
Conclusion: From this study, it is concluded that the random forests and K-NN machine leaning models are the most suitable models for breast cancer diagnosis with an accuracy rate greater than 95%.
marsbahis marsbahis mobilbahis 1xbet onwin betist piabella casino holiganbet holiganbet holiganbet mostbet mariobet meritking meritking meritking betnano casinoplus casinoplus betriyal casinoplus
Share this article
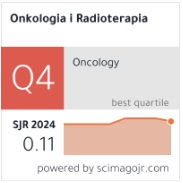
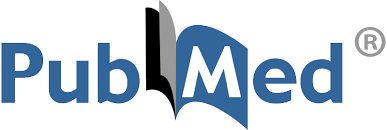
Editors List
-
RAOUi Yasser
Senior Medical Physicist
-
Ahmed Hussien Alshewered
University of Basrah College of Medicine, Iraq
-
Sudhakar Tummala
Department of Electronics and Communication Engineering SRM University – AP, Andhra Pradesh
-
Alphonse Laya
Supervisor of Biochemistry Lab and PhD. students of Faculty of Science, Department of Chemistry and Department of Chemis
-
Fava Maria Giovanna
Google Scholar citation report
Citations : 558
Onkologia i Radioterapia received 558 citations as per Google Scholar report
Onkologia i Radioterapia peer review process verified at publons
Indexed In
- Directory of Open Access Journals
- Scimago
- SCOPUS
- EBSCO A-Z
- MIAR
- Euro Pub
- Google Scholar
- Medical Project Poland
- PUBMED
- Cancer Index
- Gdansk University of Technology, Ministry Points 20