Classification of Ultrasound Breast Cancer Image Using Tuning Up the Hyper-Parameter of Convolutional Neural Network
Abstract
Author(s): Satish Bansal*, Rakesh S Jadon and Sanjay Kumar Gupta
Breast cancer in women is a significant public health concern worldwide, with many cases going undiagnosed until the advanced stages. Early detection is crucial for proper treatment and improved outcomes. There are some pre-trained models used by authors for the detection of breast tumour, but these models require extensive computation power due to their many layers and parameters. To address this issue, it is required to proposed Convolutional Neural Network (CNN) model with fewer training parameters for classification of ultrasound images dataset to determine that a particular image is either benign or malignant. In this paper, CNN model is proposed with changes in some hyper parameters like the number of filters, filter size, batch normalization, learning rate, epoch, and batch size, to achieve better accuracy with less computational power. The proposed model was compared to other pre-trained models, including ResNet50, Efficient Net, and VGG16, using two databases (database A for training and validation data, and database B for testing data). Our proposed classifier outperformed than pretrained classifiers in terms of accuracy.
Share this article
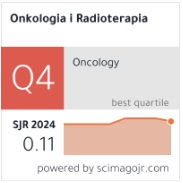
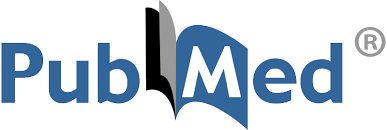
Editors List
-
RAOUi Yasser
Senior Medical Physicist
-
Ahmed Hussien Alshewered
University of Basrah College of Medicine, Iraq
-
Sudhakar Tummala
Department of Electronics and Communication Engineering SRM University – AP, Andhra Pradesh
-
Alphonse Laya
Supervisor of Biochemistry Lab and PhD. students of Faculty of Science, Department of Chemistry and Department of Chemis
-
Fava Maria Giovanna
Google Scholar citation report
Citations : 558
Onkologia i Radioterapia received 558 citations as per Google Scholar report
Onkologia i Radioterapia peer review process verified at publons
Indexed In
- Directory of Open Access Journals
- Scimago
- SCOPUS
- EBSCO A-Z
- MIAR
- Euro Pub
- Google Scholar
- Medical Project Poland
- PUBMED
- Cancer Index
- Gdansk University of Technology, Ministry Points 20